What information do you need to make a good decision? It might seem like an obvious question, but anyone who has agonized over a large purchase (like a car or a big appliance) knows how tough it can be to decide how much information is enough to take action.
On the one hand, you don’t want to make the wrong decision based on incomplete data (whether it’s a recommendation from a friend, or a relatively simple analysis). On the other, it can take a lot of time and money to really be sure you’ve got plenty of information to draw on. If you are going to buy the most reliable car model that you can afford, it may not matter how much more reliable the best option is; regardless, you’ll take the same action.
A new paper on our team’s work published in Remote Sensing in Ecology and Conservation tackles this question in a conservation context. We were doing a detailed analysis in support of the Camboriú water fund in Brazil, examining how planned conservation work would impact water quality in the river.
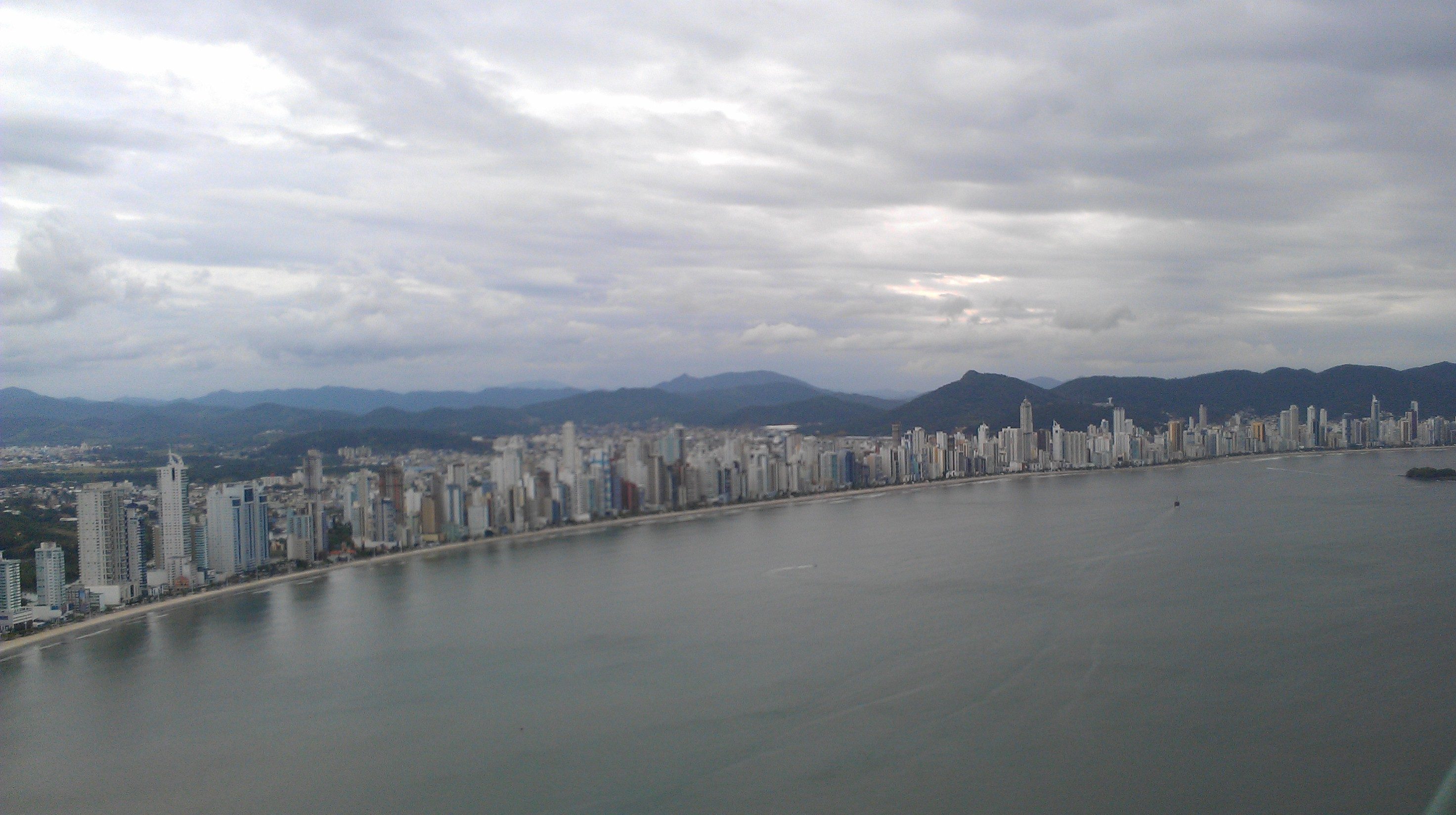
To avoid water shortages during the tourist high season, the Camboriú water treatment company had to both meet the growing demand for water and address increasing water quality problems. Their options were limited and included (1) flooding farms and forest to store more water, (2) bringing in water from a neighboring watershed (which was low quality and would require advanced and expensive treatment), or (3) adopting the water fund conservation program that The Nature Conservancy promoted (which had lower up-front investment costs, and offered co-benefits like reducing flooding and preserving biodiversity).
Making Decisions in Camboriú
The company decided to invest in conservation, but wanted to know how much it would help the water supply problems to determine whether or not the conservation solution would be sufficient to meet all of their water supply and treatment needs.
While we were conducting the detailed analysis of the impact of conservation on water quality, I noticed that each of several modeling runs took two weeks to complete, and started wondering — given that Camboriú had already decided to invest in the conservation option — whether a simpler model would have been enough.
So we compared the water quality model we had built with state-of-the-art data (custom 1m resolution satellite imagery), to a simpler model (based on free 30m imagery), which was both less costly and much faster to develop. The model only took a day to run instead of two weeks.
We found that the simpler, less expensive model would have led to the same decision in Camboriú (to invest in conservation), but that in other decision contexts the higher-resolution model could have made an important difference by providing more precise measurements and greater detail in specific areas.
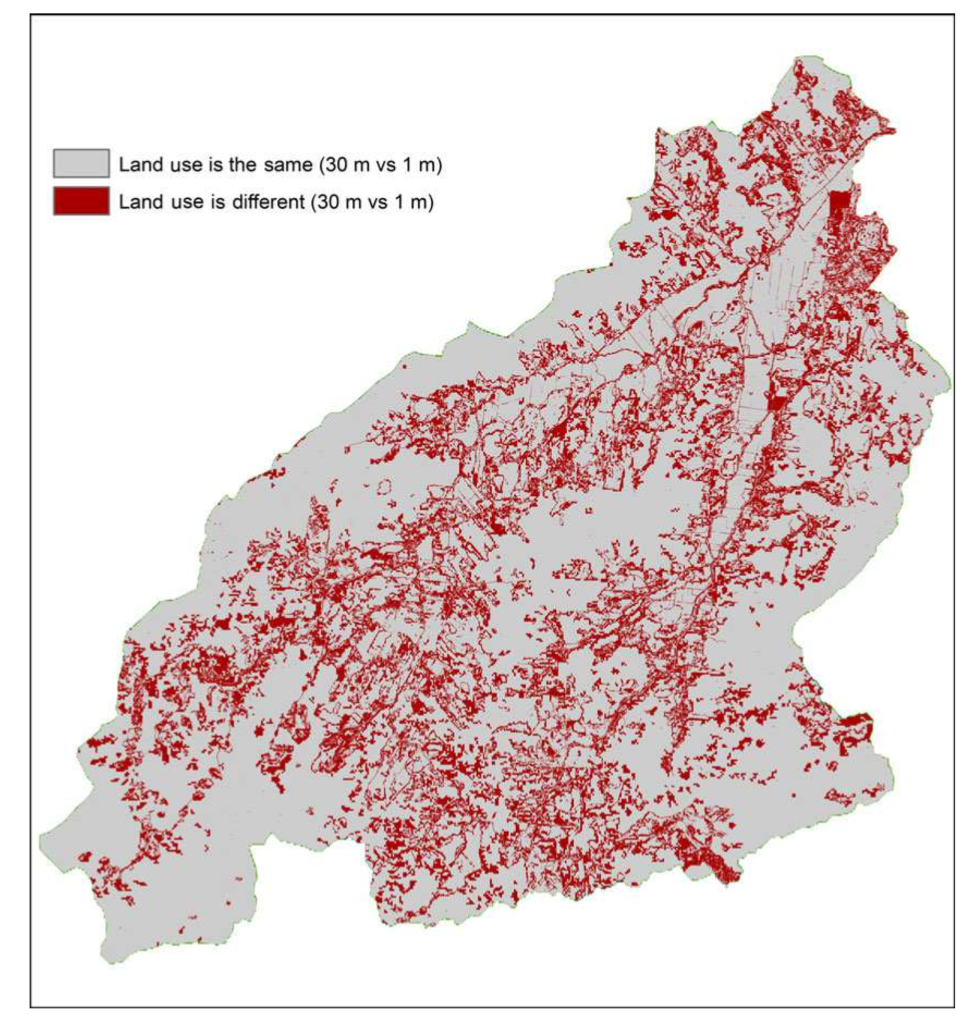
The lower-resolution model was 7 percent less accurate in terms of estimated water quality (sediment load), and it predicted 12 percent lower benefits of conservation than the higher-resolution model showed. That is a relatively small difference, but it meant that the return on investment (ROI) was over 1 for the higher-resolution model (conservation paid for itself after 44 years), but for the lower-resolution model, ROI was always below 1 (conservation looked more expensive than traditional “gray” water treatment).
This is where context comes in. In this specific case, given the co-benefits that come with conservation, and the high initial costs of the alternative solutions, the ROI being below 1 (as shown by the lower-resolution model) would not have changed the decision of the water treatment company in Camboriú. But in another water fund, where there were more options or requirements, models based on higher-resolution data would be necessary to ensure decision-makers were making choices based on the most precise, accurate information available.
Our paper offers a set of guidelines in deciding whether or not higher-resolution data may be necessary (Table 5).
For example, for a project with a large watershed that is fairly homogeneous and that will require frequent updates despite a low budget, starting with free lower-resolution data probably makes sense. But in projects where there is a low tolerance for error in the models (and a high risk of making the wrong decision based on those errors), then, especially if an initial model produces estimates close to an important decision making threshold (like an ROI close to 1), the use of higher-resolution data would be preferred.
“Best” Might Not Always be Best
It’s tempting to always go for what you think is the “best” data; and in conservation and remote sensing that often means drones or new satellites with very high resolution. But as the authors of this study have learned here and in other projects, those data sources can be expensive to acquire and time-intensive to work with, kind of like trying to read hundreds of product reviews on the internet!
With a bit of thought and planning, conservation projects should be able to save time and money with lower-resolution data in some cases, and in others identify where the extra cost is important and necessary to make the right decision.
For those interested in learning more, a new scientific paper about the overall water fund project in Brazil in December 2018: https://www.sciencedirect.com/science/article/pii/S0048969718349611?via%3Dihub
Thank you, Jon! Both your blog and paper are an important contribution to the conversation on water funds, market-based conservation in general, and uncertainty. Any comments or analyses on the level of monitoring needed (a similar comparison, e.g., between lower- and higher data requirements) to conduct adaptive management in this fund? That is, to know whether the conservation/restoration actions are enough to achieve the desired water quality or flow regulation? Thanks again.
Hi Jo, great question. In either case, I’d recommend using actual stream water quality data to answer the question of how well conservation is working. Our model performs pretty well, but during the biggest storms which can cause the worst problems it’s still not quite right. Fortunately the water treatment plant already has a couple of continuous monitoring stations (the ones we used to calibrate and validate / test our model).
I’m not sure why this showed up as a comment, but clicking the text above will take you to a slightly more technical blog focusing on the issue of choosing the right spatial data source.